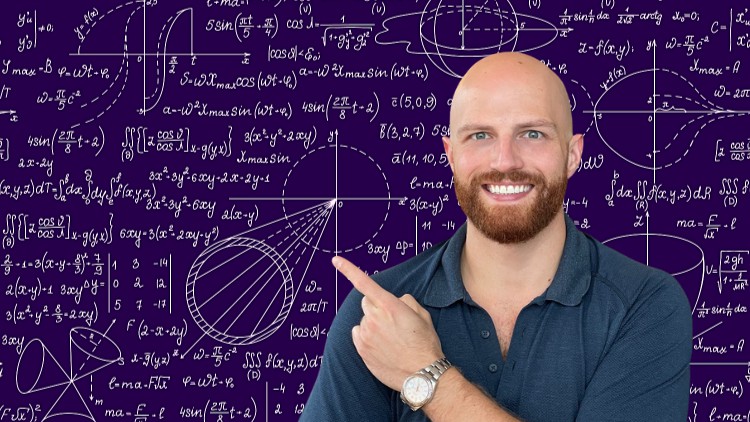
Mathematical Foundations of Machine Learning
Data science and machine learning are based on mathematics. As a result, in order to be the finest data scientist possible, you must have a solid knowledge of the most important arithmetic.
Thanks to high-level tools like Scikit-learn and Keras, getting started in data science is simple.
Understanding the mathematics behind the algorithms in these libraries, on the other hand, offers up an unlimited number of options. Understanding the arithmetic behind it all may greatly boost the effect you can have over the course of your career, from spotting modeling flaws to designing new and more powerful solutions.
This course, led by deep learning pioneer Dr. Jon Krohn, gives a solid understanding of the mathematics that underpins machine learning algorithms and data science models – specifically, linear algebra and calculus.
Sections of the Course
- Data Structures in Linear Algebra
- Tensor Operations are operations on tensors.
- Matrix Characteristics
- Eigenvalues and Eigenvectors
- Machine Learning Matrix Operations
- Limits
- Differentiation and Derivatives
- Differentiation by Machine
- Calculus of partial derivatives
- Calculus of Integrals
You’ll discover plenty of hands-on assignments, Python code demos, and practical exercises throughout each lesson to help you improve your math skills!
This course on Mathematical Foundations of Machine Learning is complete, but we want to add supplementary information from relevant areas outside of math in the future, such as probability, statistics, data structures, algorithms, and optimization.
Are you prepared to excel as a data scientist? I’ll see you in class.
visit here : Data Visualization in Python Masterclass™: Beginners to Pro